Data Scientist vs Data Analyst: Understanding the Key Differences
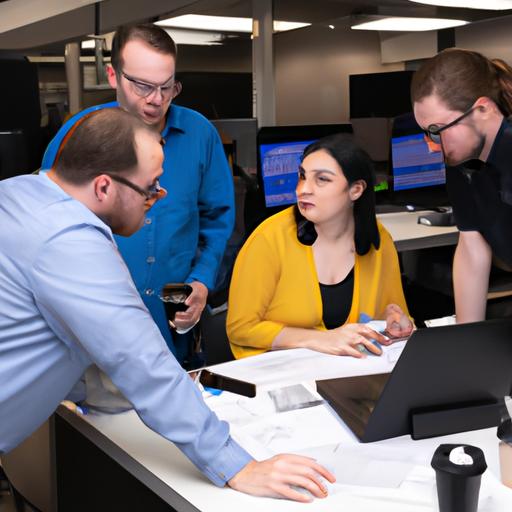
In today’s data-driven world, the terms “data scientist” and “data analyst” are often used interchangeably, leading to confusion about the roles and responsibilities associated with each. If you’ve ever wondered what sets these two professions apart, you’re in the right place. In this article, we’ll delve into the world of data science, exploring the nuances between data scientists and data analysts.
A. Definition and Importance of Data Science
Data science is the field that deals with extracting valuable insights and knowledge from vast amounts of data. It encompasses various techniques, methodologies, and tools to analyze, interpret, and visualize data, enabling organizations to make informed decisions and gain a competitive edge. With the exponential growth of data in recent years, data science has become increasingly vital across industries.
B. Distinction Between Data Scientist and Data Analyst
While both data scientists and data analysts work with data, their roles and responsibilities differ significantly. A data scientist is a multifaceted professional who possesses a strong background in mathematics, statistics, and computer science. Their primary objective is to develop complex algorithms, build predictive models, and uncover hidden patterns in data. Data scientists are skilled in programming languages like Python or R and are proficient in machine learning and artificial intelligence techniques.
On the other hand, a data analyst focuses on examining data sets to identify trends, draw conclusions, and provide actionable insights. They use statistical methods and visualization tools to present data in a meaningful way, aiding decision-making processes. Data analysts possess excellent analytical skills, proficiency in SQL, and expertise in data visualization tools like Tableau or Power B
Understanding the differences between these roles is crucial for organizations seeking to make the most of their data and talent pool. In the following sections, we will explore the specific roles and responsibilities of data scientists and data analysts in more detail, shedding light on the unique skill sets required for each profession.
Stay tuned as we dive into the world of data science, uncovering the intricacies of the data scientist and data analyst roles. By the end of this article, you’ll gain clarity on which path aligns best with your interests and aspirations. So, let’s embark on this exciting journey together!
Roles and Responsibilities of a Data Scientist
A. Overview of a Data Scientist’s Tasks and Objectives
Data scientists play a crucial role in leveraging data to drive meaningful insights and solve complex problems. Their tasks revolve around gathering, cleaning, and analyzing large datasets to extract valuable information. They are responsible for developing and implementing cutting-edge algorithms and models that can predict trends, patterns, and outcomes.
Data scientists work closely with stakeholders to understand business objectives and translate them into data-driven solutions. They collaborate with cross-functional teams, including domain experts and engineers, to create innovative solutions that address real-world challenges. Whether it’s developing recommendation systems, optimizing processes, or conducting sentiment analysis, data scientists are at the forefront of harnessing the power of data.
B. Required Skills and Qualifications for Data Scientists
To excel as a data scientist, a diverse skill set is essential. Proficiency in programming languages like Python or R is a fundamental requirement, as it enables data scientists to manipulate and analyze large datasets effectively. A solid foundation in statistics and mathematics is also crucial for developing accurate models and performing hypothesis testing.
Moreover, data scientists must possess strong problem-solving and critical-thinking abilities. They should have a deep understanding of machine learning algorithms and techniques, as well as experience with data visualization tools like Matplotlib or ggplot. Effective communication skills are also essential, as data scientists need to present their findings to both technical and non-technical stakeholders.
C. Tools and Technologies Commonly Used by Data Scientists
Data scientists employ a wide range of tools and technologies to perform their tasks efficiently. Programming languages like Python and R provide extensive libraries and frameworks specifically designed for data analysis and machine learning. These languages allow data scientists to preprocess data, build models, and visualize results seamlessly.
In addition to programming languages, data scientists utilize powerful machine learning libraries such as TensorFlow and scikit-learn. These libraries offer a plethora of algorithms and tools for building predictive models and conducting advanced data analysis. Moreover, data scientists often utilize cloud platforms like AWS or Google Cloud, which provide scalable infrastructure for processing and storing large datasets.
Data scientists also leverage data visualization tools like Tableau or Power BI to create visually appealing and interactive dashboards. These tools enable them to present complex data in a simplified manner, facilitating better understanding and decision-making.
In the next section, we’ll explore the roles and responsibilities of data analysts, shedding light on their unique contributions to the world of data science.
Roles and Responsibilities of a Data Analyst
A. Overview of a Data Analyst’s Tasks and Objectives
Data analysts play a pivotal role in organizations by transforming raw data into meaningful insights. Their primary objective is to gather, analyze, and interpret data to support decision-making processes. They work closely with various stakeholders to understand business requirements and formulate data-driven strategies.
Data analysts are responsible for performing data cleansing and validation to ensure data accuracy and integrity. They use statistical methods to identify trends, patterns, and correlations within datasets. By applying their analytical skills, they extract actionable insights from complex data, helping organizations optimize operations, improve customer experiences, and identify potential market opportunities.
B. Required Skills and Qualifications for Data Analysts
To excel as a data analyst, certain skills and qualifications are essential. Proficiency in statistical analysis, data manipulation, and data visualization are fundamental. Strong analytical thinking and problem-solving abilities are crucial to effectively analyze complex datasets and derive valuable insights.
Data analysts must be well-versed in programming languages such as SQL, Python, or R, as these are often used to query databases and manipulate data. Knowledge of data visualization tools like Tableau, Power BI, or Excel is also important to present data in a visually appealing and easily understandable manner.
Additionally, data analysts should possess excellent communication skills to effectively convey their findings to non-technical stakeholders. The ability to translate complex data into actionable insights is vital for driving informed decision-making processes within organizations.
C. Tools and Technologies Commonly Used by Data Analysts
Data analysts leverage various tools and technologies to perform their tasks efficiently. SQL, a standard database querying language, is widely used for data extraction and manipulation. Python and R are popular programming languages for statistical analysis and data manipulation, providing data analysts with extensive libraries and packages for data exploration.
Data visualization tools like Tableau, Power BI, and Excel allow analysts to create compelling visualizations and interactive dashboards to present data insights effectively. These tools facilitate the communication of complex information in a visually appealing manner.
In addition to these core tools, data analysts also work with spreadsheets, statistical software packages, and data analytics platforms, depending on their organization’s specific requirements.
Stay tuned as we move forward to compare the roles of data scientists and data analysts, highlighting their distinct skill sets and responsibilities. Understanding these differences will help you determine which path aligns best with your interests and career aspirations. So, let’s dive deeper into this exciting realm of data science!
Comparison between Data Scientists and Data Analysts
A. Scope of Work and Focus Areas
Data scientists and data analysts have distinct scopes of work and focus areas within the realm of data science.
Data scientists are primarily responsible for developing and implementing complex algorithms, predictive models, and machine learning solutions. They dive deep into data, utilizing statistical analysis and advanced techniques to uncover patterns, correlations, and trends. Their work revolves around solving complex problems, designing experiments, and applying cutting-edge technologies to extract valuable insights from data.
In contrast, data analysts focus on analyzing and interpreting data to provide actionable insights for decision-making. They work with structured and sometimes unstructured data, utilizing statistical methods, data visualization, and reporting tools. Data analysts aim to understand trends, identify patterns, and communicate findings to stakeholders effectively. Their work involves conducting ad-hoc analysis, generating reports, and supporting business intelligence initiatives.
B. Differences in Technical Skills and Expertise
Data scientists and data analysts possess different technical skills and expertise that align with their respective roles.
Data scientists require a strong foundation in mathematics, statistics, and computer science. They are proficient in programming languages like Python or R and have knowledge of machine learning algorithms, deep learning, and natural language processing. Additionally, data scientists are skilled in data manipulation, data visualization, and working with big data technologies like Hadoop or Spark.
On the other hand, data analysts excel in data querying and manipulation using SQL. They have expertise in data visualization tools like Tableau or Power BI, enabling them to create insightful dashboards and reports. Data analysts are adept at using statistical analysis techniques, such as hypothesis testing and regression analysis. Moreover, they possess strong problem-solving and critical-thinking abilities to derive meaningful insights from data.
C. Variances in Education and Training Requirements
The educational and training requirements for data scientists and data analysts differ, reflecting the specific skills needed for each role.
Data scientists typically hold advanced degrees, such as a Master’s or Ph.D., in fields like computer science, statistics, or mathematics. These programs equip data scientists with the necessary theoretical knowledge and research skills to tackle complex data challenges. Additionally, data scientists often engage in continuous learning, attending workshops, online courses, and industry conferences to stay updated with the latest advancements in the field.
In contrast, data analysts may hold a Bachelor’s degree in fields like statistics, mathematics, economics, or computer science. While a higher degree can enhance their career prospects, many data analysts gain practical skills through hands-on experience and professional certifications. These certifications often focus on data analysis, data visualization, and SQL querying, providing practical knowledge to excel in the role.
D. Potential Career Paths and Growth Opportunities
Both data scientists and data analysts have promising career paths and numerous growth opportunities within the evolving field of data science.
Data scientists can pursue various career paths, such as machine learning engineer, data engineer, or research scientist. With experience and expertise, they can advance to leadership positions, leading data science teams or driving innovation within organizations. The demand for data scientists is continuously increasing, offering them a wide range of opportunities in industries like healthcare, finance, technology, and more.
Similarly, data analysts have diverse career paths available to them. They can progress to senior analyst roles, business intelligence managers, or data analytics consultants. Data analysts have the opportunity to specialize in specific industries or domains, such as marketing analytics, financial analysis, or healthcare analytics. As organizations increasingly recognize the value of data-driven decision-making, data analysts are in high demand across industries.
Understanding the differences between data scientists and data analysts in terms of scope, technical skills, education, and career paths is essential for aspiring professionals in the field. This knowledge enables individuals to make informed decisions about their career trajectory, leveraging their strengths and interests to contribute effectively in the dynamic world of data science.
Collaborative Efforts between Data Scientists and Data Analysts
A. Importance of Cooperation and Synergy between the Two Roles
In the ever-evolving landscape of data science, collaboration between data scientists and data analysts is crucial. While their roles may differ, their collective efforts can lead to more comprehensive and impactful insights. The synergy between these professionals allows for a holistic approach to data analysis, driving innovation and problem-solving.
Data scientists bring their expertise in advanced statistical modeling and machine learning algorithms to the table. Their ability to build complex models and extract intricate patterns from data sets forms the foundation for data analysis. On the other hand, data analysts possess a deep understanding of data visualization, statistical analysis, and domain-specific knowledge. Their skills enable them to uncover meaningful insights from data and present them in a digestible format.
B. How Data Scientists and Data Analysts Complement Each Other’s Work
Data scientists and data analysts work in tandem to enhance the value and impact of data-driven initiatives. While data scientists focus on developing sophisticated models and algorithms, data analysts leverage these tools to extract actionable insights and present them in a user-friendly manner. The collaboration between these roles bridges the gap between raw data and effective decision-making.
Data scientists rely on data analysts to validate and interpret their models, ensuring that the insights generated are accurate and relevant. In turn, data analysts rely on data scientists to provide them with the necessary tools and frameworks for analysis. This symbiotic relationship ensures that the insights derived from data are robust, reliable, and align with organizational goals.
C. Examples of Successful Collaborations and Their Benefits
Numerous real-world examples highlight the power of collaboration between data scientists and data analysts. For instance, in the healthcare industry, data scientists and data analysts work together to analyze patient data, identify risk factors, and develop predictive models. This collaboration enables healthcare providers to make informed decisions, improve patient outcomes, and optimize resource allocation.
In the e-commerce sector, data scientists collaborate with data analysts to perform customer segmentation and predict purchasing behavior. By combining their expertise, these professionals can personalize marketing strategies, enhance customer experiences, and drive revenue growth.
The benefits of collaborative efforts between data scientists and data analysts extend beyond specific industries. By leveraging both technical expertise and domain knowledge, organizations can gain a comprehensive understanding of their data, leading to data-driven strategies, improved efficiency, and a competitive advantage.
In the next section, we will conclude our exploration of the data scientist and data analyst roles, summarizing the key points discussed throughout this article. Stay tuned to gain a comprehensive perspective on these professions and their significance in the realm of data science.
Collaborative Efforts between Data Scientists and Data Analysts
In the realm of data-driven decision making, collaboration between data scientists and data analysts is essential for success. Although their roles may differ, their combined efforts can unlock the full potential of data and drive impactful insights.
A. Importance of Cooperation and Synergy
Data scientists and data analysts bring unique perspectives and expertise to the table. By working together, they can leverage their respective skills to tackle complex problems and achieve more comprehensive results. The collaboration between these professionals fosters a cohesive data-driven approach, ensuring that organizations make well-informed decisions based on accurate and reliable insights.
B. Complementary Work of Data Scientists and Data Analysts
Data scientists and data analysts complement each other’s work in various ways. While data scientists focus on developing advanced models and algorithms, data analysts excel at interpreting and visualizing data. Data scientists thrive in exploring intricate patterns and building predictive models, while data analysts excel in extracting meaningful insights and presenting them in a digestible manner. It’s this symbiotic relationship that enables organizations to extract maximum value from their data.
C. Examples of Successful Collaborations and Their Benefits
Successful collaborations between data scientists and data analysts have been instrumental in solving real-world problems and driving innovation. For instance, in healthcare, data scientists can develop predictive models to identify patients at risk, while data analysts can analyze healthcare data to improve operational efficiency and patient outcomes. In e-commerce, data scientists can develop recommendation systems, while data analysts can analyze customer behavior to optimize marketing strategies. These examples illustrate how collaboration between data scientists and data analysts can drive tangible outcomes and create a competitive edge.
In conclusion, the collaboration between data scientists and data analysts is crucial for organizations to harness the full potential of their data. By leveraging their unique skills and perspectives, these professionals can work in harmony, unlocking valuable insights and driving informed decision-making. The synergy between data scientists and data analysts paves the way for innovation and growth in today’s data-driven landscape. So, embrace the power of collaboration and witness the transformative impact it can have on your organization’s data journey.
Conclusion: So above is the Data Scientist vs Data Analyst: Understanding the Key Differences article. Hopefully with this article you can help you in life, always follow and read our good articles on the website: news.mail1s.com